- What is a snowflake
- What is databricks
- Why snowflake
- Why databricks
- Key difference between snowflake vs databricks
- Key Features of Snowflake
- Key Features of Databricks
- Advantages of Snowflake
- Advantages of Databricks
- Conclusion
What is a snowflake?
Snowflake is a data warehouse tool that is cloud-based. Snowflake offers all the data warehouse functions on a single tool without the need for several system integrations. The snowflake team updated the traditional cloud data warehouse concept to make it a contemporary and fully managed cloud data warehouse.
In contrast to a legacy data warehouse, it is simple to operate, extremely affordable, and quick to scale. Data sharing and scaling are made easier and with less overhead because of decoupled storage and processing. Customers can easily load, integrate, process, analyse, and share their data which abstracts away cloud complications. Additionally, as Snowflake was created and developed from a data warehouse perspective, migrating to it is very simple.
Become a Snowflake Certified professional by learning this HKR Snowflake Training !
What is databricks
Databricks is a cloud platform that specialises in large-scale data analysis regardless of where the data is stored. It is a platform for data and analytics that aids businesses in retrieving business intelligence from data. With its Managed MLflow, collaborative notebooks, and machine learning runtime, it also offers a whole data science workspace.
Due to its capacity to process enormous amounts of data, Databricks is well known in the industry. It also supports various languages, which enhances its power by enabling the integration of libraries from any programming language ecosystem. Consequently, Large businesses utilise Databricks for the production operations across various sectors, including entertainment, healthcare, fintech, etc. A lake house architecture can be built using the open-source storage layer called Delta Lake, which is offered by Data bricks.
Why snowflake
Snowflakes are acquired by the customers for mainly 3 reasons. They are
- A better option to EDW 1.0: Who needs to spend money on large metal boxes, property investment to house them, and hiring people to manage them? No-one. Not even the CIA or the NSA.
- Snowflake, like EDW 1.0, can be a great option for business intelligence workloads, where it shines the brightest.
- Snowflake's interface is extremely simple to use. For such a purpose, it will proceed to cater to the strategist community, as it did with EDW 1.0. In the cloud, clients no longer worry regarding managing hardware. They wouldn't even have to worry about handling the software with Snowflake.
Why databricks
Databricks will help to grow customers for three primary reasons:
- Superior technology: Till we see leadership varies like Google, Netflix, Uber, and Facebook transformation from open source to hardware products, you can be confident that open-source systems like Databricks are superior in terms of technology. They are far more adaptable.
- Data science and machine learning: As with Data Lake 1.0 vs EDW 1.0, the Databricks framework is unquestionably ideally suited to data science and machine learning workforces than Snowflake.
- Minimal Vendor Lock-In: As with Data Lake 1.0, vendor lock-in is minimal, if at all, with Databricks. In fact, Databricks allows you to leave your data wherever you want. Connect to it with Databricks and procedure it for practically any use case.
Acquire Databricks certification by enrolling in the HKR Databricks Training program in Hyderabad!
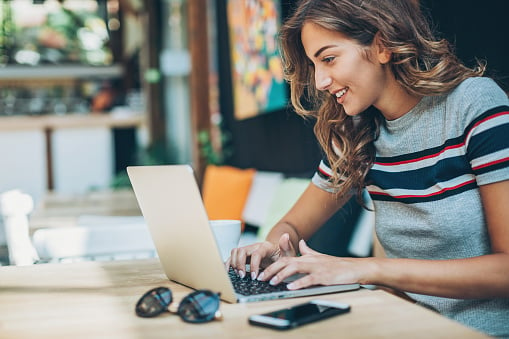
Snowflake Training
- Master Your Craft
- Lifetime LMS & Faculty Access
- 24/7 online expert support
- Real-world & Project Based Learning
Key difference between snowflake vs databricks:
Data structure:
Snowflake:Unlike EDW 1.0 and similar to a data lake, Snowflake allows you to upload and save both structured and semi-structured files without first organizing the data with an ETL tool before loading it into the EDW. Snowflake will automatically transform the data into its internal structured format once it has been uploaded. Snowflake, unlike a data lake, does require you to add structure to your unstructured data.
Databricks:Databricks, like Data Lake 1.0, can work with all data types in their original format. In fact, Databricks can be used as an ETL tool to structure unstructured data so that other tools, such as Snowflake, can work with it.
Data Ownership:
Snowflake:Snowflake would then tell users that, in comparison to EDW 1.0, the storage and processing layers have been decoupled. That is, you can scale each independently in the cloud based on your needs. This will save you money because, as we've seen, we only process about half of the data we store. Snowflake, like the legacy EDW, does not decouple data ownership. It still controls both the processing layers and data computation.
Databricks:Databricks, on the other hand, completely decouples the data storage and processing layers. Databricks is more concerned with the data processing and application layers. You can end up leaving your information anywhere it is (including on-premises) and in any layout, and Databricks will process it.
Versability:
Snowflake:Snowflake, like EDW 1.0, is particularly fit for SQL-based, Business Intelligence use cases, where it excels. Working on data science and machine learning use cases with Snowflake data will almost certainly necessitate reliance on their partner ecosystem. Snowflake, like Databricks, provides ODBC and JDBC drivers for integrating with third-party systems.These partners would most likely take Snowflake data and process it using a processing engine other than Snowflake, such as Apache Spark, before returning the results to Snowflake.
Databricks:High-performance SQL queries are also supported by Databricks for Business Intelligence use cases. Databricks developed open-source Delta Lake as an additional layer of dependability on top of Data Lake 1.0. Users now can accept SQL queries to high-performance levels normally reserved for SQL queries to an EDW using Databricks Delta Engine on top of Delta Lake.
Features:
Snowflake:Snowflake comes with database, security features, provides good support, security validations and integrations, etc.
Databricks:The features offered are collaboration, interactive exploration, databricks runtime, job scheduled, dashboards, integrated identity management, auditing, notebook workflows, etc.
Pricing:
Snowflake:Snowflake offers four enterprise based pans for the users. They are standard edition, premier edition, enterprise edition and enterprise edition for sensitive data.
Databricks:Databricks comes with three enterprise pricing options for the users. They are databricks for data engineering workloads, databricks for data analytics workloads, and databricks enterprise plans.
Integrations:
Snowflake: Snowflake can be easily integrated with the following business systems and applications such as looker, AWS, tableau, talend and fivetran, etc.
Databricks: Databricks can be integrated with the following business systems and applications such as looker, Amazon redshift, tableau, talend, pentaho, alteryx, redis, cassandra, MongoDB, etc.
Automation :
Snowflake: In order to automatically manage incoming data in close to real-time, Snowflake uses its “Snowpipe” tool. It uses auto-schema to incoming data, detects sensitive information, and divides it accordingly, resolving a major data categorization issue that businesses encounter while managing their security posture. Snowflake automatically encrypts data in transit and at rest to maintain the security of data transmission, regardless if it is sensitive or not.
Databricks: Databricks offers automation for complicated data engineering and pipeline activities. The Databricks platform automatically processes incoming data so that it is prepared for analysis. Before it is filtered for useful business insights and distributed among the users, data automatically goes through quality control to weed out inaccuracies.
Collaboration :
Snowflake: Snowflake Data Cloud makes it possible for internal and external stakeholders to securely collaborate across departments, clouds, and geographic locations. To make sure that all are on the same page, all authorised users have access to a live data set, and also they have the option for tailored access to restrict a user's view or access. On top of Snowflake's built-in security capabilities, administrators can control who has access, keep track of usage and access, and manage the publishing workflow.
Databricks: Databricks makes it easier for data engineers, data analysts and data scientists, to collaborate with the option of delta sharing. Information can be shared among various data platforms like Tableau, Power BI, etc.. Additionally, Databricks offers Unity Catalog, a native integration that enables customers to audit and manage data sharing between the organisations.
Data Lake :
Snowflake: Both Data Cloud and Data Lake options exist from Snowflake. An updated, cloud-based data warehouse called Data Cloud provides inexpensive storage with 2 to 3 times data compression. Additionally, Snowflake supports data lake architecture, which automatically collects, organises, and protects the data. It collects data in any format like structured, semi-structured, and unstructured over the clouds and locations. The elastic processing engine of Snowflake makes it possible to analyse and query data quickly and effectively, making a variety of structured data easily accessible for business insights. A pipeline can be developed quickly using SQL, Python, Scala, or another language of choice utilising Snowflake's Snowpark functionality.
Databricks: Databricks' Delta Lake, an open format storage layer unites the structured, semi-structured, streaming and unstructured data formats. Databricks' Delta Lake, which is powered by Apache Spark, enables scalability and performance. Through its Delta Live Tables, which manage data pipelines and keep them updated with fresh data, Delta Lake makes it simpler for data engineers to establish the data lake house foundation.
Machine Learning (ML) :
Snowflake: Snowflake can be used to create machine learning and analytics, however it doesn't come with these features out of the box and instead needs integration with the other solutions. But it offers drivers that let you access the data and interface with other platform libraries or modules.
Databricks: A strong machine learning environment is available at Databricks for the creation of various models. It also allows programming in a variety of languages, making it simpler to employ libraries and modules.
Although Snowflake doesn't have any ML libraries, it provides connectors to combine different ML tools. Additionally, it makes its storage layer or export query results accessible, which may be employed for testing and training of models.
SQL :
Snowflake: With the ability to handle any scale workload, Snowflake's SQL query function provides seamless data analytics. Users can select multi-cluster computing resources for almost limitless concurrency, and its provision computer clusters meet demand required for a workload. Snowflake also supports ANSI SQL and semi-structured data, in order to enable the insights users will get through their preferred BI tool. Popular BI/analytics products can be directly connected to using Snowflake's SQL capability. Insights from SQL queries can also be shared through Snowsight, a built-in visualisation tool in Snowflake.
Databricks: Data analysts may easily extract insights using SQL queries that are directly retrieved from the Databricks data lake using SQL on Databricks. The Photon query engine from Databricks enables faster queries at a cheaper cost and without requiring code modification. Through SQL endpoints on Databricks, users may leverage data insights through their preferred BI tool without experiencing any latency. Finally, in order to promote collaboration, save time, and reduce repetition, analysts can store queries and share dashboards that they build.
Scalability :
Both solutions make use of cloud computing to scale faster and with little additional cost. Snowflake is restricted to 128 nodes, whereas Databricks can be scaled to that extent you could afford in the infrastructure. Additionally, Snowflake has alternatives for fixed-sized warehouses, which allow users to easily change the size of clusters but not individual nodes. Moreover, Snowflakes provides auto-suspend and auto-scaling, allowing clusters to begin or end at busy or inactive periods. Contrarily, Databricks allows you to provision numerous node types at various size levels, although it is more difficult than a single click. In order to scale the Databricks cluster, you must possess the required technical knowledge.
Security :
Databricks provides distinct customer keys, full RBAC for clusters, pools, jobs, and table-level, and data security for customers. In contrast, Snowflake offers distinct customer keys.
Architecture :
Due to the decoupling of storage and compute, data lakes' architecture sets them apart from traditional data warehouses. Databricks offers a separate layer for computing and storage, making it easier to scale and use the various processing engines best suited for each use case.
In contrast, Snowflake features a separate storage and processing layer even though it is a managed service and its architecture is visible to end users. Databricks provides you the ability to select the appropriate node despite the fact that node types of Snowflake are unknown.
Use Cases :
The design and architecture of Snowflake make it particularly well suited for SQL-based business analytics use cases. In contrast, Databricks supports a wider range of use cases, including intrusion detection and recommendation engines, and permits SQL-based business intelligence. Additionally, dashboards for reporting and analytics are supported by both solutions.
Any high-volume system can have its throughput requirements met by Databricks, but its query speed for analytics will be subpar. Whereas, Snowflake can outperform Databricks while having limited capabilities for concurrency and continuous writes.
Get ahead in your career with our Snowflake Tutorial !

Subscribe to our YouTube channel to get new updates..!
Subscribe
Key Features of Snowflake
1. Increased Speed and Quality of Analytics :
Snowflake makes it possible for you to improve your Analytics Pipeline, By transforming from nightly batch loads to the real time data streams. By enabling secure, concurrent, and governed access to your data warehouse in your organisation, you can improve the quality of analytics at work. This reduces costs and manual labour, enabling organisations to distribute resources optimally to maximise income.
2. Better Data-Driven Decision Making :
Snowflake enables you to eliminate Data Silos and provide everyone in the organisation access to actionable insights. This is a crucial initial step in enhancing partner relationships, optimising pricing, cutting costs associated with operations, increasing sales effectiveness, and many other things.
3. Improved User Experiences and Product Offerings :
Using Snowflake, we can understand the user behaviour as well as the usage of the product in a better way which will lead to improved user experiences and product offerings. To provide customer success, greatly enhance product offers, and promote Data Science innovation, you can also use the complete data.
4. Customised Data Exchange :
With Snowflake, you may develop your own Data Exchange that enables you to safely communicate live, governed data. Additionally, it encourages you to build stronger data relationships throughout your business units as well as with your partners and clients. This is accomplished by developing a 360-degree angle of your client, that provides information on essential client characteristics like interests, employment, etc.
5. Robust Security :
All the compliance and cybersecurity data may be centralised in a secure data lake. Rapid incident response is assured by snowflake data lakes. By gathering huge amounts of log data in one place and fastly evaluating years' worth of log data, this helps you to get a clear idea of an incident. Semi-structured logs as well as structured enterprise data can be integrated in a single data lake. Without the need for indexing, Snowflake enables you to access while making it easy to manipulate and transform data when it is imported.
Without dividing the primary tasks, Snowflake enables Data Scientists and Data Analysts to explore and find new connections. For many industries, including retail, where quick information is essential for success, this is a major advantage.
Key Features of Databricks
Due to its capacity to princess and manage massive amounts of data, Databricks has established itself as an industry-leading solution for Data Analyst and Data Scientists. Here are a handful of key features of Databricks:
1. Delta Lake :
An open source and transactional storage layer for the entire data lifecycle is available in Databricks. This layer can be used to give your existing Data Lake data scalability and reliability.
2. Optimised Spark Engine :
Databricks give you access to the most latest Apache Spark releases. Numerous Open-source libraries can be easily integrated with Databricks. You can fastly set up clusters and build a completely managed Apache Spark environment if there is access to scalability and availability of various cloud service providers. Clusters may be configured, fine tuned and set up using Databricks without the need for continuous monitoring to balance optimal dependability and performance.
3. Machine Learning :
Using cutting-edge frameworks like Scikit-Learn, Tensortflow and Pytorch, Databricks gives you one-click access to preconfigured Machine Learning environments. You can also share, track and experiment, manage models together and replicate runs all from one central repository.
4. Collaborative Notebooks :
With the right tools, we may rapidly access and analyse your data, create models together and find and share useful insights. Using Databricks, we can program in any language we choose, like SQL, R, Scala and Python.
Advantages of Snowflake :
1. Implementation assistance :
Snowflake architecture is flexible and effective. Furthermore, it is frequently cited as one of the easiest data warehouses for data migration. In addition, Snowflake requires no complex IT architecture or equipment to set up or manage because it is a cloud-based data platform.
2. Cloud Initialization :
The Snowflake structure is built on the basis of cloud computing. Due to its cloud-first approach, Snowflake database servers are suitable for cross-cloud workloads as well as multi-cloud platforms. Snowflake is accessible to Both Microsoft Azure and Amazon Web Services.
3. Performance :
Snowflake's current cloud architecture allows it to avoid many of the problems that come with traditional data warehouses, which improves performance in general. Snowflake isolates concurrent workloads on dedicated resources, enabling nearly unlimited scalability. This suggests that each person, team, program, or automated task may work independently from the rest of the system without affecting how well the system functions as a whole.
4. Administration is not Required :
Snowflake doesn’t need any IT infrastructure or management as it is entirely cloud based. It guarantees that datasets of any size can have quick access and recovery because of its built in speed optimization, data security and safe data transmission features.
Advantages of Databricks
1. Languages Support :
Despite being spark-based, Databricks also supports popular programming languages like R, Python and SQL. To interface with Spark, these technologies were translated via APIs on the backend. As a result, users no longer need to learn new programming languages in order to use networked analytics.
2. Microsoft stack integration :
Azure Active Directory Architecture protects Databricks. If proper security settings are in place, the authorization of current credentials may be used. The administration of access and identities takes place in the same environment. Connectivity with the whole Azure stack along with Data Lake Storage is made simple by using Azure Active Directory.
3. Several data Sources :
In addition to Azure-based sources, Databricks links to a wide range of other resources, including those on SQL servers, CVS files and JSON files.
4. Suitable for small projects as well :
Databricks may be used for smaller projects and improvement even though it is best suited for large-scale operations. As a result, Databricks may be used as a one-stop shop for all analytics tasks. Companies are no longer required to create unique virtual machines or development environments.
Top 30 frequently asked snowflake interview questions & answers for freshers & experienced professionals
Conclusion:
With the exception of Snowflake, you could indeed collaborate with your information in a range of languages in relation to SQL. This is particularly critical for data science and machine learning applications. To work with big data, data analysts mainly use the R and Python programming languages.Databricks offers a cooperative data science and machine learning platform in addition to secure connections for these languages.
Related Article:
About Author
As a senior Technical Content Writer for HKR Trainings, Gayathri has a good comprehension of the present technical innovations, which incorporates perspectives like Business Intelligence and Analytics. She conveys advanced technical ideas precisely and vividly, as conceivable to the target group, guaranteeing that the content is available to clients. She writes qualitative content in the field of Data Warehousing & ETL, Big Data Analytics, and ERP Tools. Connect me on LinkedIn.
Upcoming Snowflake Training Online classes
Batch starts on 29th Mar 2025 |
|
||
Batch starts on 2nd Apr 2025 |
|
||
Batch starts on 6th Apr 2025 |
|
FAQ's
Due to its open-source roots, Databricks is more widely used by developers than Snowflake. Databricks is constructed using three key open-source platforms: Delta Lake, Apache Spark, and MLflow. Beyond simply SQL, it also supports a wide range of other programming languages. The benefit Databricks has inside its OS origins has been clearly recognised by Snowflake, which is much more of a closed ecosystem. Snowflake has also made a number of attempts, such as by supporting Apache Iceberg, a well-known open table format for analytics in an effort to interact with the open source community
For data science and machine learning use cases, Databricks was initially developed as a data lake based on open-source Spark. At the same time, Snowflake built a cloud data warehouse that could be utilised for business intelligence analytics. The majority of clients will utilise both Databricks and Snowflake for their areas of expertise respectively.
Organisations can utilise Databricks ETL as a data and AI solution to speed up the operation and functioning of ETL pipelines. The platform offers data management, security, and governance features and may be utilised in a number of sectors.
The platform includes scalable services to create enterprise data pipelines, so it has everything you need whether you're a data scientist, data engineer, developer, or analyst. The platform is very flexible and simple to master in around a week.
Some of the competitors of Snowflake are Amazon Redshift, MongoDB, Oracle database, Db2, Cloudera Enterprise Data Hub, etc.,