Apache Spark Tutorial
Last updated on Jun 12, 2024
Apache Spark Tutorial - Table of Content
- What is Apache Spark?
- Spark installation
- Spark Architecture
- Spark Components
- Apache Spark Compatibility with Hadoop
- Conclusion
What is Apache Spark?
Apache Spark is a lightweight open-source framework that handles the real-time generated data. It was designed to make fast computations based on Hadoop MapReduce. In other words Apache spark was developed for speeding up the Hadoop computing process. MapReduce model was extended by Apache Spark to use it more efficiently for computations that include stream processing and interactive queries. In-Memory cluster computing increases the processing speed of the application which was the main feature of Spark.
Apache Spark covers a wide range of workloads such as iterative algorithms,interactive queries,batch applications and streaming. Along with all these workloads, it reduces the burden to the management for maintaining separate tools.
Apache Spark History:
In 2009, Matei Zaharia developed Spark as one of Hadoop's sub-projects in UC Berkeley's Lab. Under a BSD license, it was open-sourced in 2010. After that, Spark was donated to Apache software foundation in 2013.Now it has emerged as a top-level Apache project.
Why should you learn Apache Spark?
The data that is being generated is increasing day by day.The traditional methods cannot access this huge volume of data. To eliminate this problem, Big data and Hadoop emerged. But they too had some limitations.These limitations can be eliminated by Apache spark. So Apache Spark has become more efficient because of its speed and less complexity.
Spark toolset is continuously expanding, which is attracting third-party interest. So boost your career by learning Apache spark from this Apache Spark Tutorial. Here you can write the applications in any of the programming languages like Java,Python, R, Scala that you are comfortable with. Moreover, Spark developers were paid high salaries.
Become a Apache Spark Certified professional by learning this HKR Apache Spark Training !
Spark installation:
Step 1: Before installing Apache Spark, we need to verify if Java was installed or not.If Java is already installed, proceed with the next step; otherwise, Download Java and install it on your system.
Step 2: Then Verify if Scala is installed in your system. If it is already installed, then proceed; otherwise, download Scala's latest version and install it in your system.
Step 3: Now, Download the latest version of Apache Spark from the following Link.
https://spark.apache.org/downloads.html
You can see the Spark Zip file in your download folder.
Step 4: Extract it. Then create a folder named Spark under user Directory and copy-paste the content from the unzipped file.
Step 5: Now, we need to configure the path.
Go to Control Panel -> System and Security -> System -> Advanced Settings -> Environment Variables
Add new user variable (or System variable)
(To add a new user variable, click on the New button under User variable for
Now, Add %SPARK_HOME%\bin to the path variable.
And Click OK.
Step 6: Spark needs Hadoop to run.For Hadoop 2.7,you need to install winutils.exe.
You can find winutils.exe from the following link. Download it
https://github.com/steveloughran/winutils/blob/master/hadoop-2.7.1/bin/winutils.exe
Step 7: Create a folder named winutils in the C drive and create a folder named bin inside. Move the downloaded winutils file to the bin folder.
C:\winutils\bin
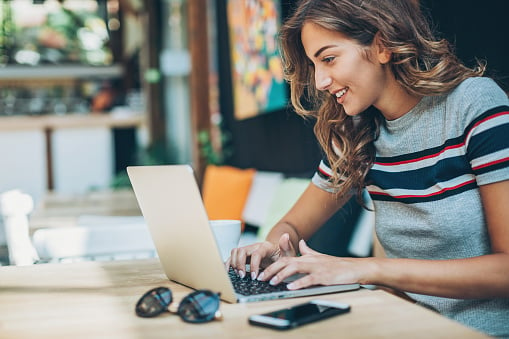
Apache Spark Certification Training
- Master Your Craft
- Lifetime LMS & Faculty Access
- 24/7 online expert support
- Real-world & Project Based Learning
Spark Architecture:
Apache Spark Architecture is a well-defined and layered architecture, where all the layers and components are loosely coupled. This Architecture is integrated with various libraries and extensions. In other words, it is said that Spark Architecture follows Master-Slave architecture, where a cluster consists of a single master and multiple workers nodes.
Apache Spark architecture mainly depends upon two abstractions:
- Directed Acyclic Graph (DAG)
- Resilient Distributed Dataset (RDD)
Top 30 frequently asked Apache Spark Interview Questions !
1. Directed Acyclic Graph (DAG):
Directed Acyclic Graph is a sequence of computations performed on data. Here each node is an RDD partition, and each edge is a transformation on top of data. DAG eliminates the Hadoop MapReduce multistage execution model and provides performance enhancements over Hadoop.
Let us understand it more clearly.
Here the Driver Program runs the main() function of the application.It creates a SparkContext object whose primary purpose is to run as an independent set of processes on the cluster and coordinate with the spark applications. So to run on a cluster, SparkContext connects with different cluster managers. Then it acquires executors on nodes in the cluster and sends the application code to the executors. Here the application code can be defined by Python or JAR files. Finally, the SparkContext sends the tasks to the executors to run.
2. Resilient Distributed Dataset (RDD):
Resilient Distributed Datasets are the collection of data items that are split into different partitions and stored in the memory of the spark cluster's worker nodes.
RDD's can be created in two ways:
- By Parallelizing existing data in the driver program and
- By referencing a dataset in the external storage system
Parallelized Collection: Parallelized collections are created by calling the SparkContext's parallelize method on an existing driver program collection. The elements of the collection are copied to form a distributed dataset that can be operated in parallel.
Here is an example of how to create a parallized collection holding the numbers 1 to 3.
val info = Array(1, 2, 3)
val distnumbr = sc.parallelize(numbr)
External Datasets: From any storage sources supported by Hadoop such as HDFS, HBase, Cassandra, or even the local file system, distributed datasets can be created. Spark supports text files, Sequence Files, and any other Hadoop InputFormat.
To create RDD's text file, SparkContext's textfile method can be used. URI for the file is taken by this method, either a hdfs:// or a local path on the machine, and reads the file's data.
Example invocation:
scala> val distFile = sc.textFile("data.txt")
distFile: org.apache.spark.rdd.RDD[String] = data.txt MapPartitionsRDD[10] at textFile at:26
distFile can be acted on by dataset operations once it is created. For example, Sizes of all the lines can be added using map and reduce operations.
distFile.map(s => s.length).reduce((a, b) => a + b).
RDD Operations: RDD provides two types of Operations. They are:
- Transformation
- Action
i) Transformation:
In Spark, the role of Transformation is to create a new dataset from an existing one. As they are computed when an action requires a result to be returned to the driver program, the transformations are considered lazy.
Some of the RDD transformations that are frequently used are:
- map(func) - It returns a new distributed dataset formed by passing each element of the source through the function func.
- filter(func) - It returns a new dataset formed by selecting those elements of the source on which func returns true.
- flatMap(func) - It is similar to map, but each input item can be mapped to 0 or more output items. (Therefore, func should return a Sequence rather than a single item).
- mapPartitions(func) - It is similar to map, but runs separately on each partition (block) of the RDD. Therefore func must be of type Iterator
=> Iterator while running on an RDD of type T. - mapPartitionsWithIndex(func) - It is similar to mapPartitions, but it also provides func with an integer value representing the partition index. So func must be of type (Int, Iterator
) => Iterator while running on an RDD of type T. - sample(withReplacement, fraction, seed) - Using a given random number generator seed, It samples a fraction fraction of the data, with or without replacement.
- union(otherDataset) - It Returns a new dataset that contains the union of the elements in the source dataset and the argument.
- intersection(otherDataset) - It returns a new RDD that contains the intersection of elements in the source dataset and the argument.
- distinct([numPartitions])) - It returns a new dataset that contains the distinct elements of the source dataset.
- groupByKey([numPartitions]) - When called on a dataset of (K, V) pairs, it returns a dataset of (K, Iterable
) pairs. Using reduceByKey or aggregateByKey will yield much better performance if you are grouping in order to perform an aggregation (such as a sum or average) over each key. To set a different number of tasks, You can pass an optional numPartitions argument. - reduceByKey(func, [numPartitions]) - When called on a dataset of (K, V) pairs, it returns a dataset of (K, V) pairs where the values for each key are aggregated using the given reduce function func which must be of type (V,V) => V.
- aggregateByKey(zeroValue)(seqOp, combOp, [numPartitions]) - When called on a dataset of (K, V) pairs, it returns a dataset of (K, U) pairs where the values for each key are aggregated using the given combine functions and a neutral "zero" value.
- sortByKey([ascending], [numPartitions]) - When called on a dataset of (K, V) pairs where K implements Ordered, it returns a dataset of (K, V) pairs sorted by keys in ascending or descending order as specified in the boolean ascending argument.
- join(otherDataset, [numPartitions]) - When called on datasets of type (K, V) and (K, W), it returns a dataset of (K, (V, W)) pairs with all pairs of elements for each key. Outer joins are supported through rightOuterJoin, leftOuterJoin and fullOuterJoin.
- cogroup(otherDataset, [numPartitions]) - When called on datasets of type (K, V) and (K, W), it returns a dataset of (K, (Iterable
, Iterable )) tuples. - cartesian(otherDataset) - When called on datasets of types T and U, it returns a dataset of (T, U) pairs (all pairs of elements).
- pipe(command, [envVars]) - It pipes each partition of the RDD through a shell command, e.g., a bash or Perl script.
- coalesce(numPartitions) - It decreases the number of partitions in the RDD to numPartitions.
- repartition(numPartitions) - It reshuffles the RDD data randomly to create either more or fewer partitions and balances it across them.
- repartitionAndSortWithinPartitions(partitioner) - It repartitions the RDD according to the given partitioner and, within each resulting partition, sort records by their keys.
ii) Action:
In Spark,the role of action is to return a value to your driver program after running a computation on the dataset.
Some of the RDD actions that are frequently used are:
- reduce(func) -It aggregates the elements of the dataset using a function func that takes two arguments and returns one. In order to compute it correctly in parallel, the function should be commutative and associative.
- collect() - At the driver program, it returns all the elements of the dataset as an array. This is usually useful either after a filter or other operation that returns a small subset of the data.
- count() - It returns the number of elements in the dataset.
- first() - It returns the first element of the dataset.
- take(r) - It returns an array with the first r elements of the dataset.
- takeSample(withReplacement, num, [seed]) - It returns an array with a random sample of num elements of the dataset, with or without replacement.
- takeOrdered(r, [ordering]) - It returns the first r elements of the RDD using either their natural order or a custom comparator.
- saveAsTextFile(path) - It is used to write the dataset elements as a text file in a given directory in the local filesystem, HDFS, or any other Hadoop-supported file system. To convert it to a line of text in the file, Spark calls toString on each element.
- saveAsSequenceFile(path) - It is used to write the dataset elements as a Hadoop SequenceFile in the given path in a local filesystem, HDFS or any other Hadoop-supported file system.
- saveAsObjectFile(path) - It is used to write the dataset elements in a simple format using Java serialization, which can then be loaded using SparkContext.objectFile().
- countByKey() - It is available only on RDDs of type (K, V). It returns a hashmap of (K, Int) pairs with the count of each key.
- foreach(func) - It runs a function func on all the dataset elements for side effects such as updating an Accumulator or interacting with external storage systems.
Subscribe to our YouTube channel to get new updates..!
RDD Persistence: One of the important capabilities Spark provides is persisting a dataset in memory across operations. While persisting an RDD, each node stores in memory any partition of it that it computes and reuses in other actions on that dataset. This makes the future actions much faster. persist() or cache() methods can be used to mark an RDD to be persisted. Cache() is considered as fault-tolerant. It means, if any partition is lost, it will be recomputed automatically using the transformations that were originally created. There are different storage levels to store persisted RDD's. These Storage levels are set by passing a StorageLevel object(Scala, Java, Python) to persist(). While the Cache() method is used for the default storage level StorageLevel.MEMORY_ONLY.
Set of Storage Levels are as follows:
- MEMORY_ONLY - It is the default level that stores the RDD as deserialized Java objects in the JVM. If the RDD doesn't fit in memory, some of the partitions will not be cached and recomputed whenever they're needed.
- MEMORY_AND_DISK - RDD is stored as deserialized Java objects in the JVM. If the RDD doesn't fit in memory, it stores the partitions on the disk and reads them from there when they're needed.
- MEMORY_ONLY_SER - It stores RDD as serialized Java objects( i.e., per partition, one-byte array). It is generally more space-efficient than deserialized objects.
- MEMORY_AND_DISK_SER - It is similar to MEMORY_ONLY_SER but split partitions that don't fit in memory to disk instead of recomputing them.
- DISK_ONLY - It stores the RDD partitions only on disk.
- MEMORY_ONLY_2, MEMORY_AND_DISK_2 - It is the same as the levels above but replicates each partition on two cluster nodes.
- OFF_HEAP (experimental) - It is similar to MEMORY_ONLY_SER but stores the data in off-heap memory.
RDD Shared Variables: Whenever a function is passed to a Spark operation, it is executed on a remote cluster node and works on separate copies of all the function variables. These variables are copied to each machine, and no updates of the variables on the remote machine are propagated back to the driver program.
Spark provides two limited types of variables: Broadcast variables and accumulators.
i) Broadcast variable: Broadcast variables allow the programmer to keep a read-only variable cached on each machine rather than providing a copy of it with tasks. To reduce communication costs, Spark attempts to distribute broadcast variables using efficient broadcast algorithms. Through a set of stages, Spark actions are executed, separated by distributed "shuffle" operations. Spark broadcasts the common data required by the tasks within each stage automatically. The data broadcasted in this way is cached in serialized form and deserialized before running the task.
Broadcast variable v is created using call SparkContext.broadcast(v).
scala> val v = sc.broadcast(Array(1, 2, 3))
scala> v.value
ii) Accumulators: Accumulator is a variable that is used to perform associative and commutative operations such as sums or counters. Numeric type accumulators are supported by Spark. To create a numeric accumulator value of Long or Double type, use SparkContext.longAccumulator() or SparkContext.doubleAccumulator()
scala> val a=sc.longAccumulator("Accumulator")
scala> sc.parallelize(Array(2,5)).foreach(x=>a.add(x))
scala> a.value
Spark Components:
Spark Project consists of different components that are tightly integrated.To its core, It is a computational engine that can distribute, monitor, and schedule multiple applications.
- Spark Core: It is the heart of Apache Spark that performs the core functionality. It holds the components for task scheduling, interacting with storage systems, fault recovery, and memory management.
- Spark SQL: On the top of Spark Core, Spark SQL is built, supporting structured data. Spark SQL allows querying the data using SQL(Structured Query Language) and HQL(Hive Query Language). It also supports data sources like JSON, Hive tables, and Parquet. Spark SQL also supports JDBC and ODBC connections.
- Spark Streaming: It supports Scalable and faults tolerant processing of streaming data. To perform streaming analytics, it uses Spark Core's fast scheduling capability. It performs RDD transformations on the data by accepting data in mini-batches. Its design ensures that the applications written for streaming data can be reused with little modifications.
- MLib: It is a Machine Learning Library which consists of various machine learning algorithms. They include hypothesis and correlation testing, regression and classification, clustering, and principal component analysis.
- GraphX: It is a Library which is used to manipulate graphs and perform graph-parallel computations. It facilitates creating a directed graph with arbitrary properties that are attached to each vertex and edge. It also supports various operations like subgraph, joins vertices, and aggregate messages to manipulate the graph.
Apache Spark Compatibility with Hadoop:
Spark cannot replace Hadoop, but it influences the functionality of Hadoop. From the beginning, Spark reads data from and can write data to Hadoop Distributed File System(HDFS). We can say that Apache Spark is a Hadoop-based data processing engine which can take over batch and streaming overheads. So running Spark over Hadoop provides more enhanced functionality.
We can use Spark over Hadoop in 3 ways: Standalone, YARN, SIMR
In Standalone mode, We can allocate resources on all the machines or on a subset of machines in the Hadoop cluster. We can also run Spark side by side with Hadoop MapReduce.
Without any prerequisites we can run Spark on YARN. Spark in Hadoop stack can be integrated and use the facilities and advantages of Spark.
With Spark in MapReduce(SIMR), we can use Spark Shell in a few minutes after downloading. Hence it reduces the overhead of Deployment.
Apache Spark Uses:
Spark provides high performance for both batch data and streaming data. It is an easy to use application which provides a collection of libraries. Moreover the following are the uses of Apache Spark:
- Data Integration
- StreamProcessing
- Machine Learning
- Interactive Analysis
Related Article What is Apache Spark !
Conclusion:
There is a good demand for the expert professionals in this field. Hope this tutorial helped you in learning Apache Spark. In this tutorial, we have covered all the topics that are required to enhance your professionals skills in Apache Spark.
Apache Certification Tutorial
Apache Web Server is open-source web server creation, arrangement and the board programming. At first created by a gathering of programming developers, it is presently kept up by the Apache Software Foundation. Apache Web Server is intended to make web servers that can have at least one HTTP-based site. Prominent highlights incorporate the capacity to help different programming language, server-side scripting, a validation component and database bolster.
Become a Apache Cassandra Certified professional by learning this HKR Apache Cassandra Training !
Apache web server is utilized for facilitating sites. It is an amazing web server and has a ton of points of interest when contrasted with other web servers. You can utilize it in the two windows and Linux servers. With LAMP condition, you can setup sites and host it on your server.
Apache is a well known open-source, cross-stage web server that is, by the numbers, the most prominent web server in presence. It's effectively kept up by the Apache Software Foundation.
Notwithstanding its fame, it's additionally one of the most established web servers, with its first discharge the distance in 1995. Numerous panels have use Apache today. Like other web servers, Apache controls the off camera parts of serving your site's records to guests.
Become a Apache Ambari Certified professional by learning this HKR Apache Ambari Training !
Other Artcles:
About Author
Ishan is an IT graduate who has always been passionate about writing and storytelling. He is a tech-savvy and literary fanatic since his college days. Proficient in Data Science, Cloud Computing, and DevOps he is looking forward to spreading his words to the maximum audience to make them feel the adrenaline he feels when he pens down about the technological advancements. Apart from being tech-savvy and writing technical blogs, he is an entertainment writer, a blogger, and a traveler.
Upcoming Apache Spark Certification Training Online classes
Batch starts on 25th Jul 2025 |
|
||
Batch starts on 29th Jul 2025 |
|
||
Batch starts on 2nd Aug 2025 |
|