Snowflake Vs BigQuery - Table of Content
- What Is Snowflake?
- Why use Snowflake?
- Snowflake Data Warehouse
- What is Bigquery?
- Why use BigQuery?
- Snowflake Vs BigQuery
- Snowflake Advantages
- BigQuery Advantages
- Conclusion
What Is Snowflake?
Snowflake is a wholly managed SaaS and a cloud-based data warehousing platform, enterprise-ready software. We can flexibly run Snowflake on cloud service providers like Google Cloud, Amazon AWS, Azure, etc. It runs wholly on the public cloud infrastructure and supports data warehousing, data engineering, creating data apps, etc.
It is prevalent for its data-sharing feature. Snowflake architecture includes the following key components:- Cloud Services, Database Storage, and Query Processing. The major benefit of using Snowflake for users is that there is no need to engage people to install and manage it and to spend money to set it up. However, the Snowflake solution divides the compute functions and storage, enabling clients to use and pay for them individually.
Moreover, we can connect Snowflake with other services in different ways. It includes web-based UI, command-line clients, and drivers (ODBC & JDBC) through BI and ETL tools.
Why use Snowflake?
Snowflake is very fast, flexible, and offers user-friendly features to use. It is a popular enterprise-ready software that uses an SQL database engine with a cloud-based architecture. Also, it overcomes the features of many traditional data warehouses. We can also use Snowflake as a data warehouse and a data lake. The data cloud feature allows us to connect and collaborate locally and globally to discover new insights.
Moreover, Snowflake is an ideal solution for many companies because it offers great speed with superb performance. It provides faster processing of queries with automatic query optimization techniques. Also, it offers a user-friendly interface for both technical and non-tech people. Like traditional data warehouses, there are no administrative costs required for managing Snowflake.
It is why this SaaS platform is trendy among many giant companies across the globe.
Snowflake Data Warehouse:
Snowflake is a multi-cloud data platform in the truest sense. They can provide their customers with high availability and secure data across three clouds and multiple regions. Snowflake is available on Amazon Web Services, Microsoft Azure, and Google Cloud Platform. With Snowflake, you have a technology solution to build a scalable, highly resilient cloud environment with the agility your business requires while delivering valuable results.
Because of Snowflake's unique architecture and the cloud's flexibility, customers can use Snowflake across a wide range of use cases and workloads.
Snowflake began as a Data Warehouse, but as the company's ability to manage more and more data types grew, customers began to use Snowflake as a SQL Data Lake.
Customers could also use the Snowflake Data Exchange to firmly access content within their organizations as well as with various data partners. This significantly improves their own datasets, allowing them to run more sophisticated and powerful Data Analytics besides Data science use cases.
Become an Snowflake Certified professional by learning Snowflake Online Training from HKR trainings!
What Makes Snowflakes Unique?
Snowflake seems to have a multi-cluster, shared information architecture, which means that, like BigQuery, their storage and compute layers are separated. This allows them to instantly scale up or scale down in response to pressure without affecting performance.Micro-partitioning is used in their architecture. This implies they can work with semi-structured and structured data. So that they can handle JSON, Parquet, and other formats natively within Snowflake, and at an infinite scale.
Delivered as a service: This makes it highly simple to use and requires almost no management. Once your information is in Snowflake, they handle the rest; no need to identify, replant, or otherwise manage it, enabling people to focus on the worth in one's data.
Get ahead in your career with our Snowflake Tutorial !
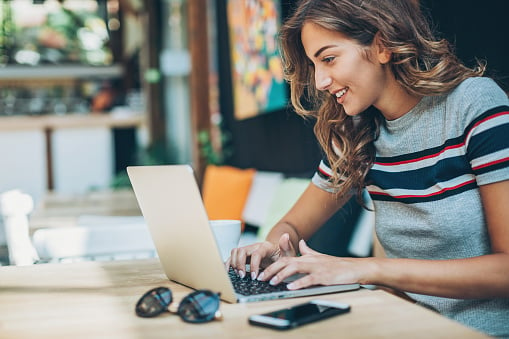
Snowflake Training
- Master Your Craft
- Lifetime LMS & Faculty Access
- 24/7 online expert support
- Real-world & Project Based Learning
What is BigQuery?
BigQuery is also an enterprise-ready data warehouse from Google, which is serverless, the most cost-effective, and has multi-cloud features. It helps to analyze and manage data using many in-built features such as ML, BI, geospatial analytics, etc. Further, it also separates the computing and storage platforms. Many large-scale companies use it, such as P&G, CNA, Toyota, UPS, etc.
Moreover, Google BigQuery comes with an in-built query engine feature that runs massive in a fraction of a second. We can get this speedy performance without the need to rebuild indexes. It analyzes billions of rows of data using a syntax similar to SQL. It is used in a Dremel form inside the Google platform. It helps track device installation data, spam check, and build crash reports.
It is also similar to Snowflake, which doesn't need to set up and manage infrastructure. Moreover, it is completely based on Google and doesn't support or run on any other platform.
Why Use BigQuery?
BigQuery is Google's serverless data warehousing platform that helps you achieve unmatched performance. It also includes some features similar to Snowflake, such as faster performance, a serverless data warehouse, provides real-time analytics with fast streaming, high availability with security, and holds a flexible pricing structure.
Without much effort, it provides capabilities like faster data exploration and analysis. It also isolates the computing and storage for better performance.
Subscribe to our YouTube channel to get new updates..!
Snowflake Vs BigQuery:
Choosing the best data warehouse for your company's needs and goals is a critical component of your big data strategy. We can see that these two data warehouses are closely stacked because they both have extensive feature sets.In terms of functionality, the leading industry standard TPC Benchmark shows little difference between Snowflake vs BigQuery. They both provide limitless concurrency and total elasticity. As a result, we chose to score this primarily on cost.
Architecture
Snowflake
Snowflake is a fully serverless solution with cloud-based data warehousing infrastructure. It isolates the storage and computing from each other. Further, its architecture is hybrid, and it is a multi-clustered shared data system. It delivers all the entity's needs, such as high performance, elasticity, scaling, etc.
Snowflake uses a central repository system that helps to make your data available throughout the compute nodes in the system. Moreover, the data inserted into Snowflake is reinvented, optimized and abridged to make a columnar form. The same is stored in the cloud storage.
Furthermore, it manages various aspects of data storage like file size, metadata, statistics, format, etc. We can only access them using SQL queries. Also, Snowflake uses virtual data warehouses to process the data.
BigQuery
It is Google's serverless data warehousing system with cost-effective features. It shares some features that are equal to the Snowflake platform. But the architecture system of BigQuery is something different than Snowflake. It uses a set of multi-tenant services which are based on popular Google-driven technologies like Dremel, Jupiter, Colossus, etc. BigQuery uses the Dremel technology, a vast multi-tenant computing cluster for the computing process. It helps to execute various SQL queries within BigQuery.
Moreover, Google's global repository system "Colossus" helps store data and manages data recovery, replication, and data management. It ensures that users don't rely on any point of failure in the system. Further, the hardware system's complete interpretation and resource distribution is handled by Borg, Google's pioneer in the Kubernetes tool. Google's Jupiter also helps in faster data movement across locations.
Pricing
Snowflake
The pricing models of Snowflake are based on each warehouse's usage. Therefore, its charges are wholly based on the total usage of the data storage system. Further, it has different types of warehouses, which broadly vary based on size and cost. Snowflake's standard edition costs are calculated on a one-credit/hour basis, where the cost per credit is USD 2. But depending on the business, the costs may vary. The cost of credit consumption may increase with the increasing size of the warehouse. Snowflake's upfront costs are comparatively lower than the on-demand cost. So, it is preferred by most entities.
BigQuery
The pricing model of BigQuery is something different from Snowflake. Google's BigQuery offers a flat-rate pricing system with an on-demand pricing option. It charges for the number of bytes it scans or reads. Also, the on-demand pricing system of BigQuery includes USD 5/TB for the number of bytes scanned or processed. On the other side, the flat rate system is followed by the slots we buy to execute the queries. It costs around USD 2000/100 slots.
Performance
Snowflake
Performance-wise, both Snowflake and BigQuery are similar. Snowflake outperforms with faster performance and querying times. It loads massive volumes of data from on-premise to cloud space quickly with faster loading. It uses the command "COPY" to load data into Snowflake. Also, it rapidly transforms broad raw data into meaningful queries. Further, it reduces the latency time for each query by speeding up the querying process.
BigQuery
It is a strong competitor of Snowflake in terms of performance. BigQuery holds several dedicated hard drives that can read around 1TB of data within a fraction of a second. Query performance optimization helps entities minimize costs. Also, it improves query speed to enhance performance. By purchasing more reserved slots, we can benefit from improved query performance.
Administration
Snowflake
Snowflake doesn't impose huge admin costs compared to others. It is designed to execute with minimal supervision of a Snowflake Admin. Auto-scaling is the essential feature that enhances or decreases the size of a virtual data warehouse. It is based on its existing workload.
BigQuery
BigQuery, similar to Snowflake, also allows us to manage various permissions, roles, and data security. Its automatic performance tuning system allows us to deal with large data volumes. With the growing data volume, the queries have also become much more complex. By addressing the various needs, it automatically scales each platform. Therefore, it manages all the maintenance automatically.
Management and Maintenance
Snowflake
Snowflake allows us to manage data processing and storage quickly, and makes solutions faster, easy usage, and more highly flexible than traditional methodologies. An advanced SaaS data platform powers it. Further, it effectively manages the data security and encryption of the stored data. Also, it maintains complete certifications relating to data warehousing, such as DSS, HIPAA, etc. It manages all the data on cloud infrastructure with full scalability and elasticity.
BigQuery
It also allows us to manage data repository, processing, solution development, and flexibly use the platform. Moreover, Bigquery manages different data types more efficiently and centrally manages, monitors, and controls data across warehouses and data lakes with remarkable consistency. It also offers easy maintenance of services.
Data Protection
Snowflake
When it comes to protecting crucial business data, both Snowflake and BigQuery perform well. Snowflake offers two distinct features to protect data: Fail-Safe and Time-travel. Time travel is used to secure the position of the data before it gets updated. There is a one-day general holding time frame for Time travel, whereas the enterprise edition allows mentioning 90 days time frame. On the other side, using the Fail-safe feature, Snowflake tries to recover the historical data which is lost or damaged. It immediately begins when the Time travel period ends.
BigQuery
In the case of BigQuery, the admins can make changes to data without worrying about recovery. It generally manages a seven-day history of all the data changes within a table. Moreover, to keep data for more than seven days, it uses table snapshots to protect data.
Security
Snowflake
Security is the central part of any data warehouse, without which no one can store or recover data safely. Snowflake allows the most trusted features for data security. It secures all the inserted data into the tables using AES encryption. All the files are auto-encrypted through the AES encryption system. Further, it provides granular permissions for schema, views, objects, tables, processes, etc., rather than individual columns.
BigQuery
It also has a set of rules for data security where it supports OAuth 2 and a multi-factor validation system similar to Snowflake. It is used for authorized access to accounts. It also provides federated user access through the Microsoft Active Directory (MAD). Further, it provides permissions only to datasets and not to individual tables or views.
Ease of Use
Snowflake
It is highly recognized for its ease of use and intuitive interface. We can immediately start using the service without disturbing the other tasks and automatically compute clusters of different sizes for any user. Also, it ensures the delivery of the performance that an organization expects.
BigQuery
Being a serverless platform, BigQuery automatically supplies additional computing resources on a required basis. By doing so, it manages massive data workloads. All this makes it easier to process massive amounts of data in just a few minutes.
Scalability
Snowflake
It offers an auto-scaling and auto-suspend feature that allows clusters to start or stop the process from managing workloads dynamically. Through this feature, users can resize the clusters with a single click. Further, we can autoscale upto 10 data warehouses within a single table.
BigQuery
It also performs similarly to Snowflake, which supplies additional computing resources based on need. Also, it manages everything in the background. Therefore, it processes large-scale data and improves scalability. Further, it allows 100 parallel users by default.
Compliance
Snowflake
Snowflake is highly dedicated to meeting regulatory compliance needs to provide better customer service. It ensures data integrity, control, and security. It uses ITAR compliance to support its customers, especially in the US region. However, Snowflake meets the compliance needs for HIPAA, PCI DSS, SOC 1 & 2 Type II, FedRamp Moderate, etc.
BigQuery
BigQuery also meets the compliance needs of HIPAA, PCI DSS, SOC 1 & 2 Type II, etc. Since BigQuery is a Google native, it provides the benefits of Google Cloud Services with proper authentication and built-in security. Therefore, it makes various integrations much more effortless.
Top 30 frequently asked snowflake interview questions & answers for freshers & experienced professionals.
Snowflake Advantages:
- Snowflake is an ANSI SQL database and data warehouse in one. As a result, they are an excellent starting point for Legacy Data Warehouses and Data Platforms looking to migrate to the cloud. They are extremely compatible with multi-statement transactions and complex joins.
- Consumers can detach workloads from across organizations and allow different departments and applications to use Snowflake. As a result, the platform can support data scientists, executive reporting, data analysts, and program managers all within the same platform while maintaining a single source of truth.
- Query concurrency is practically infinite. While using Snowflake, you could indeed scale up as needed, and when that requirement is no longer needed, Snowflake will instantly scale down. All of your customers will have direct exposure to all of the data they require at the same time.
- Queries on semi-structured data with high performance. Snowflake provides quick access to JSON, AVRO, ORC, and Parquet data, allowing for a more comprehensive view of your business and customers, allowing for deeper, more revealing insights.
- Scale up, down, and out elastic material without interfering with running queries. When the scheme is idle, there are no compute charges.
- Pricing for per-second compute and cost-effective compressed data storage.
- Snowflake offers an intuitive, user-friendly interface that helps to load and process data much faster.
- It has an excellent multi-cluster architecture that resolves various issues quickly.
- The architecture enables us to share data with many data customers flawlessly.
- It is a cost-effective platform that offers separate charges for both computing and storage.
- Snowflake supports XML, JSON, and many other data formats and addresses various issues of contrary data types.
BigQuery Advantages:
- Managed storage: One of BigQuery's main advantages is its managed storage. BigQuery provides long-term and persistent storage for your Data Warehouse, allowing you to drastically reduce data operations. Tables are saved in a columnar format that is optimized for storage. Every table has been compressed and encrypted.Streaming ingestion will be supported for all BigQuery tables. Because each table is replicated across multiple data centers, BigQuery storage is long-lasting and consistent.
- BigQuery does away with resource constraints: Their cloud-powered parallel processing query service can read from 100,000 disks simultaneously using thousands of CPUs. There is also an isolation of storage and compute to avoid scaling bottlenecks.
- BigQuery accepts a wide range of data ingestion formats: ORC, CSV, JSON, Avro Parquet.When maximizing load speed in BigQuery, use the Avro format in your ETL processes. Avro is a binary row-based format that can be split and read by multiple worker nodes in BigQuery.
- BigQuery can use nested and reiterated fields for: Tightly-coupled or immutable relationships, simplifying queries.
- Predictive Analytics with ML and GIS: BigQuery has strong AI/ML capabilities and supports a wide range of analytical use cases by utilizing:AutoML Tables – For issues involving best-in-class precision. This characteristic is completely automated and will discover the perfect model for the problem. It has a code-free graphical user interface.BigQuery ML: For problems requiring rapid experiments and development time, such as Logistic Regression, K-means, Naive Bayes, and so on. It has a SQL interface and AutoML tables as a model type.
- Faster Set up:- It allows setting up a data tool much faster for the business entities without much burden and saves much time. Also, we can start the data querying immediately after setting up the data warehouse.
- Flawless Scaling:- Scaling is a major flaw for many organizations while making data input. The companies need help managing the data size well so that it makes some sense. Google's BigQuery makes scaling much more effortless and flawless. With the division of computing and storage, the scaling becomes elastic and results in higher performance.
- Data Protection:- Data protection is essential for any business to scale and survive. It maintains a robust security layer to protect the business data and helps effectively in cases like disaster recovery.
- Cost-effective -: It is highly affordable for every business user, with flexible charges based on usage.
Conclusion:
Snowflake and BigQuery both seem to have a lot of things going for them. Both have a relatively inexpensive burden, and expenses are determined according to how much computing power and processing you require. To evaluate what cloud data warehouse is best for your organization, conduct testing in your own data consuming data and operating reports. Choosing one over the other entails determining which solution produces the most.Snowflake and BigQuery, as with most modern cloud data warehouse platforms, offer a free and proof-of-concept assistance to help companies make direct experience with how their methods deliver value.
Related Articles:
About Author
As a senior Technical Content Writer for HKR Trainings, Gayathri has a good comprehension of the present technical innovations, which incorporates perspectives like Business Intelligence and Analytics. She conveys advanced technical ideas precisely and vividly, as conceivable to the target group, guaranteeing that the content is available to clients. She writes qualitative content in the field of Data Warehousing & ETL, Big Data Analytics, and ERP Tools. Connect me on LinkedIn.
Upcoming Snowflake Training Online classes
Batch starts on 20th Apr 2025 |
|
||
Batch starts on 24th Apr 2025 |
|
||
Batch starts on 28th Apr 2025 |
|
FAQ's
Snowflake and Google's BigQuery are similar in many ways. Both are modern cloud data warehousing platforms with many tools and capabilities that help enterprise data to scale higher.
Both Snowflake and BigQuery offer relatively cheap storage costs. Snowflake charges:- For Upfront- 23 USD/TB/Month, and for on-demand- 40 USD/TB/month. Google's BigQuery charges 20 USD/TB/Month for active users and 10 USD/TB/Month for long-term users.
In Snowflake, we can store raw data within S3, allowing data access through external tables. BigQuery is a SaaS platform and a cloud data warehouse that doesn't need to manage infrastructure. Also, it uses additional computing resources to manage massive amounts of data.
BigQuery is a serverless and fully-managed cloud data warehousing platform of GCP. It helps to analyze one TB of data within a few seconds.
Snowflake replace is a widely used string function for string manipulation. The replace function deletes all the events of a specific substring. Sometimes, it, by choice, restores them with other strings.